
Will Auto-IRA Programs Affect Medicaid Enrollment?
The brief’s key findings are:
- State auto-IRA programs require employers without a retirement plan to auto-enroll their workers in an IRA.
- As participants build up IRA assets, they may end up ineligible for means-tested Medicaid when they retire.
- The analysis projects that today’s younger workers would end up with meaningful assets.
- But the effects on Medicaid may be muted, as older cohorts will have lower balances and some younger cohorts may “spend down” to get on Medicaid.
- Alternatively, states could adapt their Medicaid asset tests to exclude all, or part of, auto-IRA savings – a step already taken by California.
Introduction
Given that only about half of private sector workers are covered by an employer-sponsored plan at any given time, 16 states have launched – or are in the process of launching – auto-IRA programs that require employers without a plan to auto-enroll their workers in a Roth IRA. While these programs will help those without an employer-sponsored plan to accumulate assets for retirement, they could also put participants at risk of losing out on means-tested benefits in retirement. This risk is most frequently discussed in terms of Medicaid, which provides medical and long-term care for low-income families and where a couple’s assets generally cannot exceed $3,000.
This brief, which is based on a recent study, explores the extent to which low- and moderate-income households will not qualify for Medicaid in retirement because of their auto-IRA savings.1 Since the state auto-IRA programs are still too immature to observe participant outcomes directly, this analysis uses a simulation model to project how much auto-IRA savings future households would have if a national program had launched in 2019.
The discussion proceeds as follows. The first section provides background on the coverage gap, state auto-IRA initiatives, and Medicaid for older households. The second section describes the simulation methodology, while the third section presents the results. The final section concludes that while younger cohorts will accumulate meaningful assets in their auto-IRAs, the effect on Medicaid participation will be muted because balances for older cohorts remain low and some younger cohorts of auto-IRA participants would probably choose to “spend down” to qualify for Medicaid’s long-term care benefits.
Background
A major obstacle to boosting workers’ retirement saving is the lack of consistent access to an employer-based plan, and this coverage gap has persisted for decades. Although it particularly affects lower-wage workers without a college degree, a substantial share of even college graduates lack employer coverage at any given moment (see Figure 1).
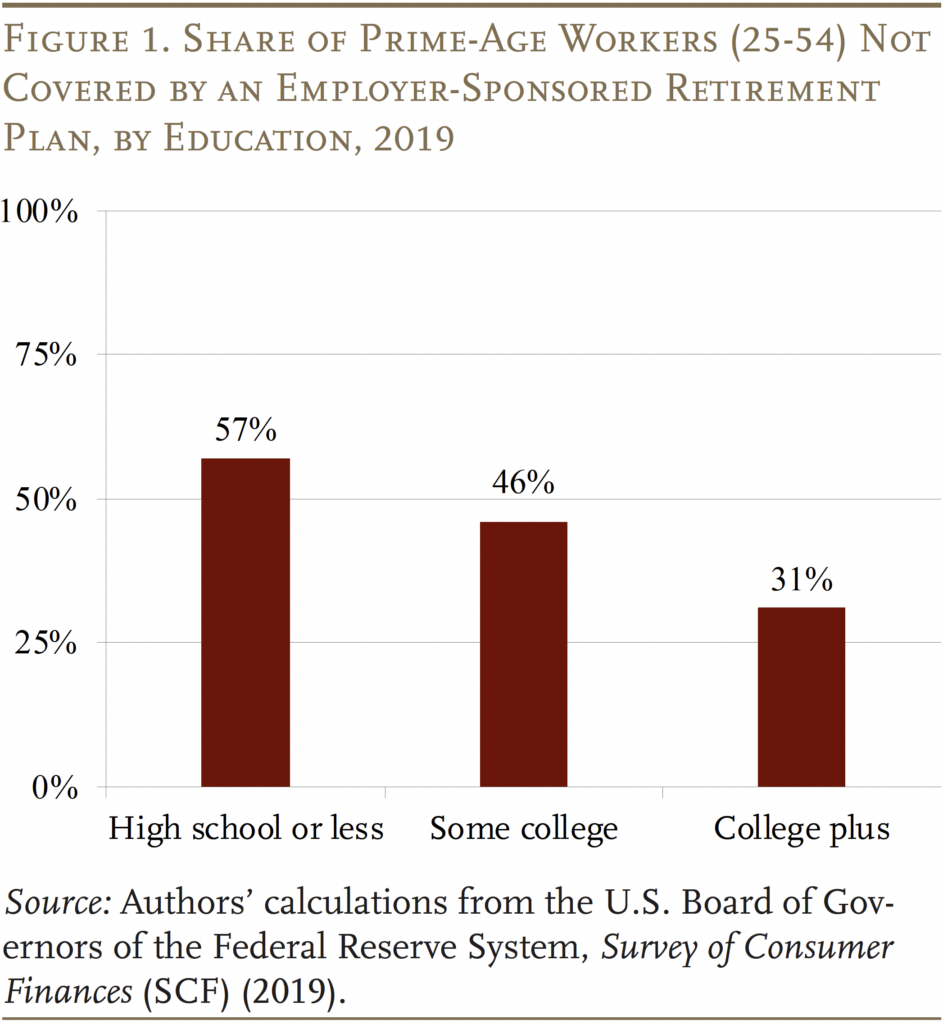
As repeated federal attempts to close the coverage gap have not had much impact, states have started implementing auto-IRA programs. Most of the existing programs follow a very similar model. Firms are required to submit timely payroll records to the program but have no fiduciary or administrative responsibility and cannot make matching contributions. Participant contributions are initially set at 5 percent with workers allowed to change the rate or opt out at any point. The first $1,000 of contributions is invested safely, with additional contributions defaulted into a target date fund. And, because the accounts are designed as Roths, workers can always withdraw their contributions with no penalty.
Although the state auto-IRAs are still in their infancy, early data on participation look promising. Early studies of OregonSaves, the first program to launch, find that participation ranged from 48 to 67 percent in 2018 (depending on the treatment of missing administrative data), suggesting that many workers did not opt out.2 More recent data provided by the live programs show opt-out rates around 30 percent.3
In terms of withdrawals, the early Oregon studies found that 20 percent of active participants made withdrawals each year, removing $1,000 from their accounts on average. However, withdrawal rates and amounts across the live programs are clearly increasing as programs extend their initial rollout to small employers and employees become increasingly aware of the option.4 Another benchmark is total withdrawals as a share of total contributions, which is currently about 25 percent in the live programs.5 The question is, will the auto-IRA arrangement translate to substantial new assets in the long run?
While asset accumulation is the goal of the auto-IRA initiative, it also raises the question whether auto-IRAs will affect the ability of older individuals to participate in means-tested programs. This concern is particularly relevant to Medicaid, which includes an asset test for those over age 65 as well as an income test. The rules vary by state and type of Medicaid services, but generally financial assets cannot exceed $2,000 for an individual and $3,000 for a couple. IRA wealth is typically included, and where it is excluded, withdrawals often count toward the income limit.6 Auto-IRA participants with assets in excess of the Medicaid thresholds may either miss out on receiving means-tested benefits or be forced to draw down their savings to preserve access.7
Methodology and Data
To determine whether low- and middle-income households are at risk of losing Medicaid benefits due to their auto-IRA savings requires projecting future balances for hypothetical workers. The projections are based on the assumption that a national IRA program had been implemented in 2019, and the analysis uses data from two sources: the Survey of Consumer Finances (SCF) and administrative data from the live auto-IRA programs. The SCF is a nationally representative triennial survey conducted by the Federal Reserve Board that provides detailed information on household assets, liabilities, income, and demographics. The SCF also has an extensive module on retirement plan coverage and participation.8 To project auto-IRA balances, the analysis also relies on opt-out rates, contribution rates, and withdrawal rates provided by the live auto-IRA programs.9
The analysis proceeds in three steps. The first step is to project lifetime employment and earnings for households in the SCF. Employment is estimated using a regression equation where the probability of being employed in future years is a function of age, gender, education, race, and their interactions. Then employed individuals are assigned earnings based on the median for current workers with similar characteristics.
The second step is to determine which employed individuals are covered by employer-sponsored plans, such as 401(k)s. Since the results turn out to be quite sensitive to this determination, the analysis is conducted under two assumptions. The first approach assigns individuals’ 401(k) coverage randomly each year, based on age-specific probabilities that vary according to gender, education, and race (the “intermittent coverage scenario”). Intuitively, this approach assumes that individuals switch jobs every year and have some chance of obtaining employer coverage at each job change.10 In contrast, a second approach assigns individuals’ lifetime 401(k) coverage randomly, at the beginning of one’s working life, based on the average coverage rate for prime-age workers of similar gender, education, and race (the “continuous coverage scenario”). Conceptually, this approach assumes that some workers always have an employer plan while others always lack one and are instead in an auto-IRA.11
The third step is to calculate auto-IRA balances. The analysis assumes that each individual who is not covered by an employer plan is eligible for the auto-IRA, and makes a one-off decision to stay auto-enrolled or to opt out. The probability of opting out is set at 30 percent, reflecting general experience from the live auto-IRAs. The employee contribution rate is set at 5 percent of earnings. Participants make withdrawals with a probability of 20 percent each year. When participants make a withdrawal, they remove $2,000 from their account, consistent with behavior in the live programs.12 The first $1,000 of contributions is held in cash; amounts in excess of $1,000 are invested in a target date fund.13
Results
To check that the model produces reasonable outcomes, Table 1 compares average auto-IRA account balances in the first few years of the projection against average balances observed in the first few years of the live programs. Reassuringly, the model seems reasonably close to real-world experience. Any deviation can be explained by the fact that we explicitly chose not to incorporate real-world complications, such as employer delays in submitting payroll records and higher-than-normal withdrawals during the COVID-19 pandemic.14
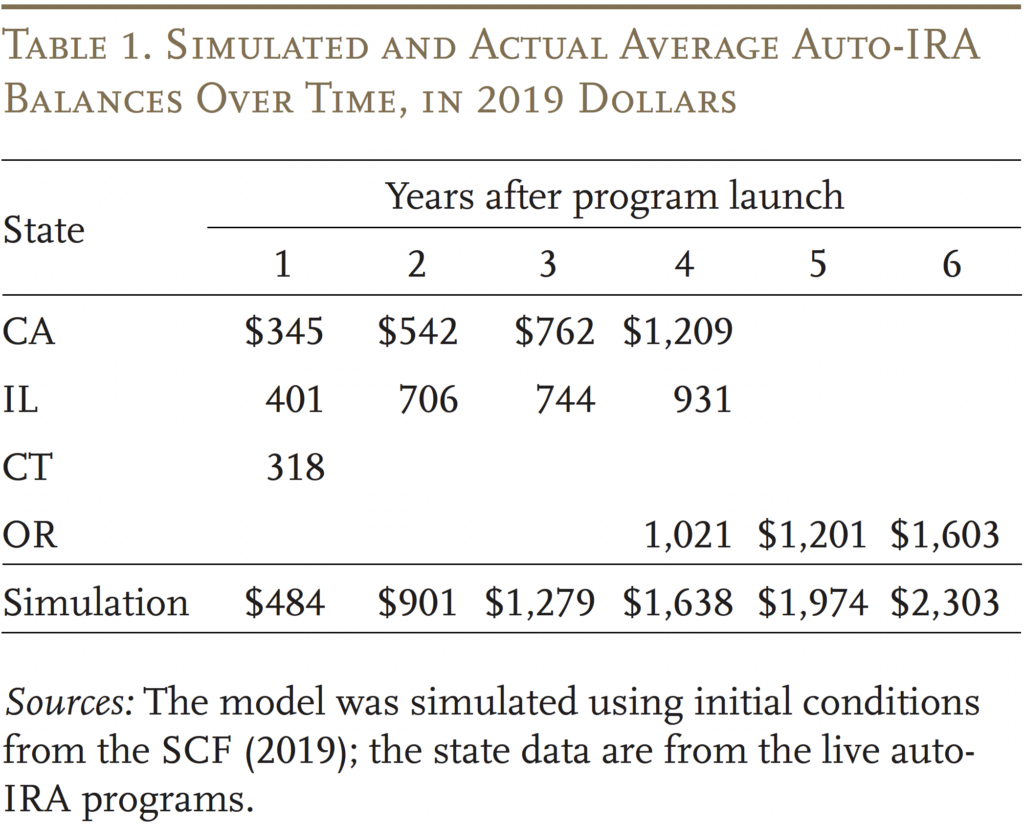
Turning to the main findings, the top panel of Table 2 shows simulation results from the “intermittent coverage” scenario at ages 51-56 for a cohort of younger workers (ages 21-25 in 2019). Since we assume that auto-IRA legislation is enacted in 2019, results for this cohort represent the highest possible balances in our model. The first column shows that about 70 percent of workers will ultimately end up with a positive auto-IRA balance. However, because participants cycle in-and-out of the program, median ending balances are moderate: $25,371 for those with no more than a high school degree, $27,595 for those with some college, and $22,304 for those with a college degree (column 2). The last column shows that the balances would be larger if participants were not also using the accounts for precautionary savings throughout their work lives.
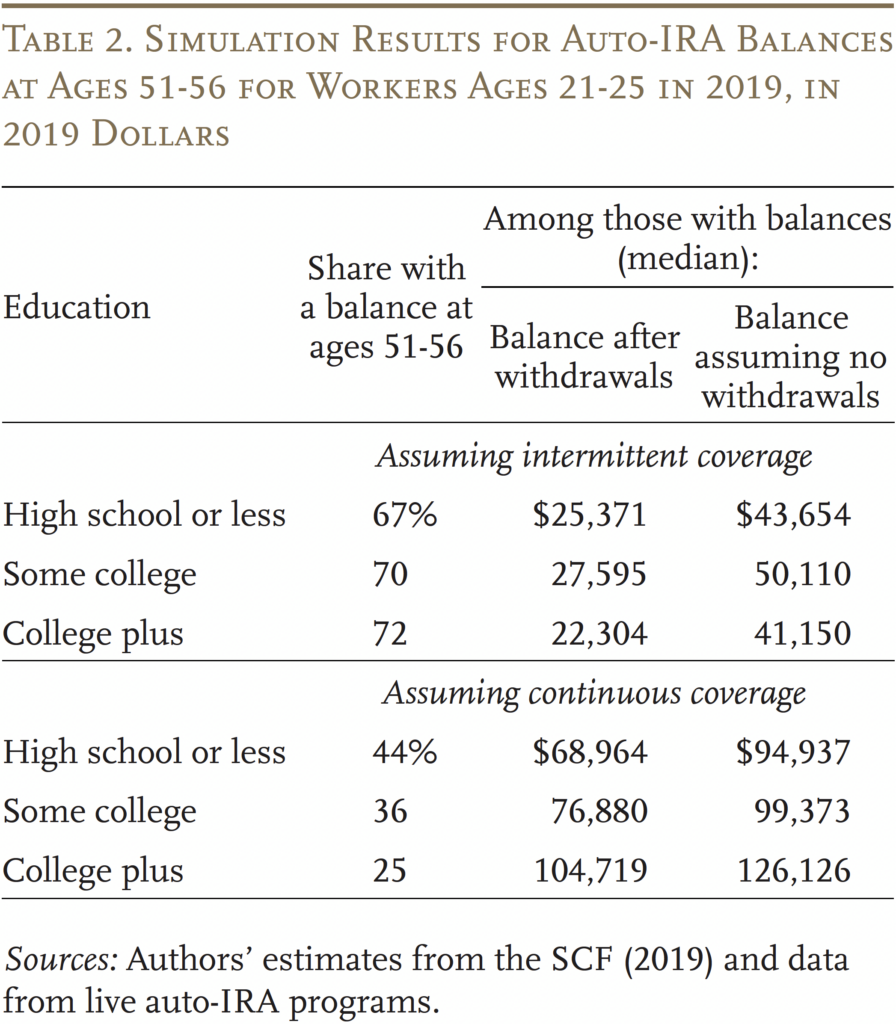
The story is different in the “continuous coverage” scenario (the bottom panel of Table 2). Here, fewer workers end up with positive balances – 44 percent of those with a high school degree or less, 36 percent of those with some college, and only 25 percent of college graduates. Yet, because participants spend their full careers in the program, they accumulate sizeable balances: $68,964 for individuals with a high school degree or less, $76,880 for those with some college, and $104,719 for college-educated workers. Of course, both the intermittent and continuous coverage scenarios are based on extreme assumptions – “intermittent coverage” underestimates the persistence of employer coverage over the work life, while “continuous coverage” overestimates it – so the most likely amounts would fall somewhere between the two.
Meanwhile, Table 3 replicates this analysis for a cohort of older workers (ages 41-50 in 2019). The share of individuals with a positive auto-IRA balance at ages 51-56 falls in the “intermittent coverage” scenario because older workers are more likely to have employer-plan coverage to begin with. Moreover, since most of these workers have relatively few years before retirement to accumulate auto-IRA savings, they end up with much smaller balances in both scenarios. Specifically, median balances after withdrawals are $4,000 to $5,000 in the “intermittent coverage” scenario, and only $8,000 to $15,000 in the “continuous coverage” scenario.
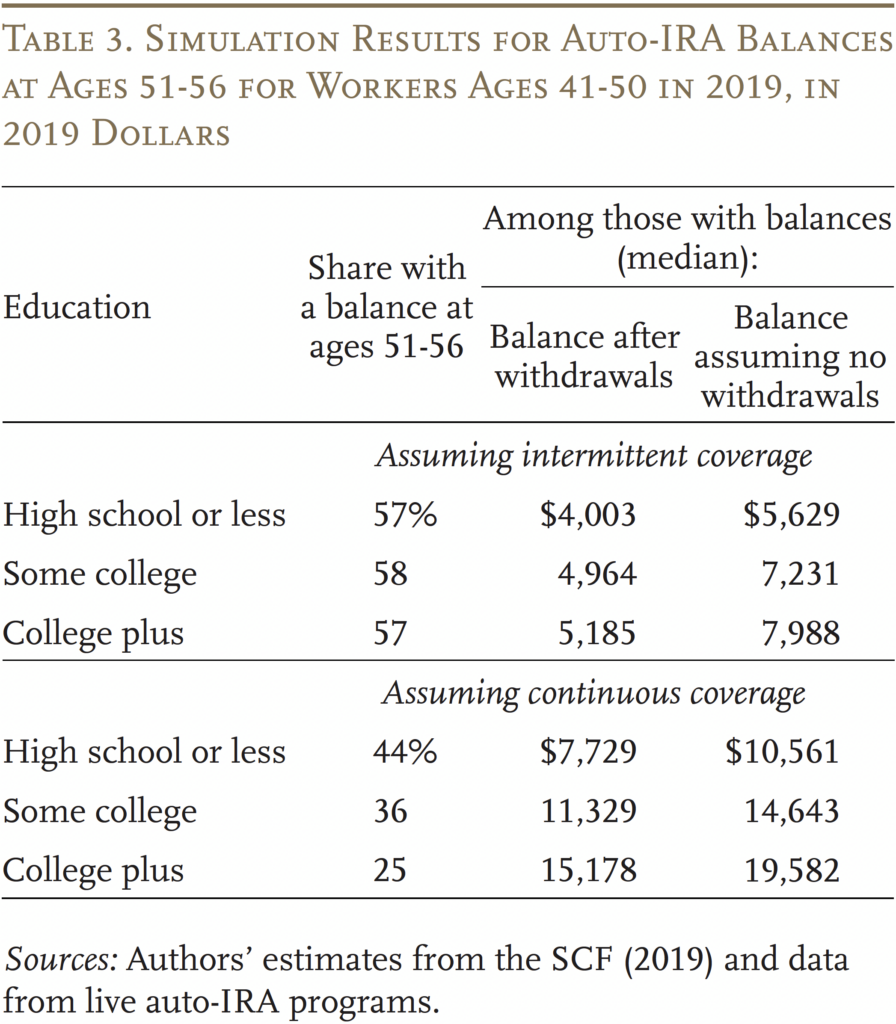
Overall, these findings suggest that, in the long run, workers accumulate significant new savings in the auto-IRA. Yet, simulated balances remain low for many years while the hypothetical program matures.
Ultimately, assessing whether these new savings will induce participants to delay or forgo Medicaid is a judgment call beyond the mechanics of the model, as it depends on participants’ post-retirement drawdown behavior and the nature of their care needs. Individuals will weigh the relative value of spending down their auto-IRA to qualify for Medicaid against preserving their balances and paying for their medical care out-of-pocket. Those who would have relied on Medicaid to subsidize their Medicare premiums or cover relatively low intensity care for a year or so will probably pay out of pocket. Those who require high intensity care for a number of years will probably spend down their own resources and then qualify for Medicaid. Not all the adjustment must come from individuals, however, as states could also change their eligibility requirements. As of January 2024, California – a state with a major auto-IRA program (CalSavers) – eliminated the asset test for older households.
Conclusion
State auto-IRA programs aim to help low and moderate earners without an employer retirement plan build savings. However, the programs’ interaction with means-tested safety-net programs, such as Medicaid, has not been discussed. This study starts the conversation by projecting what households might have saved in an auto-IRA had a national program launched in 2019. Although the results are sensitive to underlying assumptions, the analysis suggests that in the long run, workers most likely to utilize safety net programs would accumulate meaningful new savings over the course of their working lives.
Ultimately, assessing whether this new savings will cause households to delay or forgo Medicaid is a judgment call beyond the mechanics of the model. Younger cohorts of workers who have time to build up sizeable auto-IRA balances might delay Medicaid recipiency for a period. But, under current Medicaid policy, older cohorts with smaller balances will have a strong incentive to spend down their savings to qualify.
Alternatively, states could adapt their Medicaid asset tests to disregard all, or some portion of, auto-IRA savings. Beyond providing health benefits, this type of disregard might also encourage households to save more in the auto-IRA to begin with. California has already adopted such a policy, eliminating the asset test completely for those 65 and over.
References
Arapakis, Karolos and Laura D. Quinby. 2024. “Will Auto-IRA Programs Affect Medicaid Enrollment?” Working Paper 2024-14. Chestnut Hill, MA: Center for Retirement Research at Boston College.
Aubry, Jean Pierre. 2024. “A Massachusetts Auto-IRA Program.” Special Report. Chestnut Hill, MA: Center for Retirement Research at Boston College.
Belbase, Anek, Laura D. Quinby, and Geoffrey T. Sanzenbacher. 2020. “Auto-IRA Rollout Gradually Speeding Up.” Issue in Brief 20-5. Chestnut Hill, MA: Center for Retirement Research at Boston College.
California State Treasurer. 2023. “CalSavers 2023 Reports.” Sacramento, CA: CalSavers Retirement Savings Board.
Chalmers, John, Olivia S. Mitchell, Jonathan Reuter, and Mingli Zhong. 2022. “Do State-Sponsored Retirement Plans Boost Retirement Saving?” AEA Papers and Proceedings 112: 142-146.
Chetty, Raj, John N. Friedman, and Emmanuel Saez. 2013. “Using Differences in Knowledge Across Neighborhoods to Uncover the Impacts of the EITC on Earnings.” American Economic Review 103(7): 2683-2721.
Georgetown Center for Retirement Initiatives. 2024. “State Program Performance Data – Current Year.” Washington, DC.
Harris, Timothy F., Kenneth Troske, and Aaron Yelowitz. 2018. “How Will State-Run Auto-IRAs Affect Workers?” Journal of Retirement 6(2): 27-33.
Illinois State Treasurer. 2023. “Secure Choice Performance Dashboards.” Springfield, IL.
Musumeci, MaryBeth, Priya Chidambaram, and Molly O’Malley Watts. 2019. “Implications of the Expiration of Medicaid Long-Term Care Spousal Impoverishment Rules for Community Integration.” Issue Brief. San Francisco, CA: KFF.
Oregon Retirement Savings Board. 2023. “Monthly OregonSaves Program Data Reports.” Salem, OR: Oregon State Treasury.
Quinby, Laura D., Alicia H. Munnell, Wenliang Hou, Anek Belbase, and Geoffrey T. Sanzenbacher. 2020. “Participation and Pre-Retirement Withdrawals in Oregon’s Auto-IRA.” Journal of Retirement (Summer 2020): 1-13.
Sabelhaus, John. 2022. “The Current State of U.S. Workplace Retirement Plan Coverage.” Working Paper No. 2022-07. Philadelphia, PA: Wharton Pension Research Council.
Saez, Emmanuel. 2010. “Do Taxpayers Bunch at Kink Points?” American Economic Journal: Economic Policy 2(3): 180-212.
Scott, John and Andrew Blevins. 2020. “Oregon State Retirement Program Growing During Pandemic – Despite Some Worker Withdrawals: Program Assets and Participation by Employees and Employers Continue to Rise.” Washington, DC: The Pew Charitable Trusts.
U.S. Board of Governors of the Federal Reserve System. Survey of Consumer Finances, 2019. Washington, DC.
Endnotes
- Arapakis and Quinby (2024). ↩︎
- Chalmers et al. (2022); Quinby et al. (2020); and Harris, Troske, and Yelowitz (2018). ↩︎
- California State Treasurer (2023); Illinois State Treasurer (2023); and Oregon Retirement Savings Board (2023). Opt-out rates after the initial start-up periods and up to 2023 were around 30 percent overall; in 2024, opt-out rates have been somewhat higher in Illinois and California. ↩︎
- California State Treasurer (2023); Illinois State Treasurer (2023); and Oregon Retirement Savings Board (2023). ↩︎
- Georgetown University Center for Retirement Initiatives (2024). ↩︎
- Musumeci, Chidambaram, and Watts (2019). The Supplemental Security Income program counts IRA wealth in its asset limit and withdrawals as income. ↩︎
- Chetty, Friedman, and Saez (2013) and Saez (2010) show how low earners adjust earnings to qualify for programs. ↩︎
- The overall coverage rates reported in the SCF are close to the “gold standard” rates based on the Internal Revenue Service’s Statistics of Income data (see Sabelhaus 2022). ↩︎
- Some of these data are publicly available on program websites; others were provided directly to the authors (see, for example, Quinby et al. 2020 and Aubry 2024). ↩︎
- This approach understates disparities across socioeconomic (SES) groups because most simulated individuals end up with some employer coverage over the course of their working life. ↩︎
- This approach overstates disparities across SES groups as, in practice, some workers cycle in-and-out of coverage. ↩︎
- Arapakis and Quinby (2024). ↩︎
- The analysis assumes stock returns of 7 percent and bond returns of 4 percent per year. In terms of fees, the auto-IRA is assumed to charge $24 dollars and 0.45 percent of total assets per year. The target date fund also charges a fee of 0.59 percent. While consistent with the fee structure of current state programs, fees would likely be lower in a national program operating at scale (Aubry 2024). ↩︎
- Belbase, Quinby, and Sanzenbacher (2020) and Scott and Blevins (2020). ↩︎