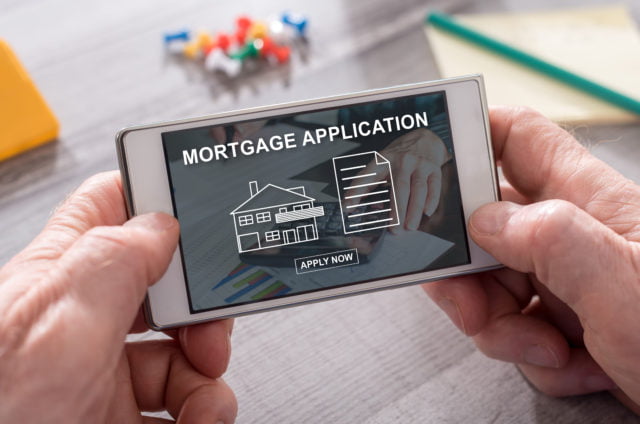
Are Older Mortgage Applicants More Likely to Be Rejected?
The brief’s key findings are:
- As the population ages, an important question is whether aging affects a person’s ability to access credit.
- This study explores the question using data on mortgage refinance applications from the confidential Home Mortgage Disclosure Act.
- The analysis, which controls for a host of applicant, property, and loan characteristics, finds that rejection rates rise substantially with age.
- A potential explanation is mortality risk, which increases the likelihood of the loan being paid off early or entering foreclosure.
- Thus, the results do not necessarily indicate that lenders are violating fair lending rules.
Introduction
As the U.S. population ages and lifespans increase, it is important to understand how aging affects an individual’s ability to access credit. Older homeowners tend to have more financial resources and better credit scores than their younger counterparts, so one would expect that they could borrow more easily. But is that true?
This brief, which is based on a recent paper, is the first of a two-part series that looks at the relationship between age and mortgage outcomes.1Amornsiripanitch (2022). This initial brief uses confidential Home Mortgage Disclosure Act (HMDA) data to study the relationship between age and the probability of being denied a mortgage application. The second brief will examine the relationship between age and the interest rate charged on mortgages.
The discussion proceeds as follows. The first section briefly outlines the extent to which a borrower’s age can legally be considered in credit decisions. The second section describes the data, which focus on rate-and-term refinance mortgages for single applicants, and the methodology, which relates the probability of rejection to age and a host of control variables. The third section presents the results, which show that rejection rates rise consistently with age. And the magnitude is large; applications for the three oldest age groups are 1-3 percentage points more likely to be rejected than those of younger applicants. These numbers equal or exceed the marginal rejection rates for Black and Hispanic applicants. The fourth section offers some possible reasons for this relationship: the underwriters frequently cite “insufficient collateral,” but the underlying issue may be mortality risk, which is tightly associated with prepayment, default, and recovery risks.
The final section concludes that while the relationship between age and rejection is large and robust, it does not necessarily indicate that lenders are violating fair lending legislation. First, the regulations allow lenders to consider borrower’s age under some circumstances. Second, although the equation controls for many observable characteristics, the results should be viewed as an association between age and rejection – not a causal relationship. Third, mortality risk has real economic implications for lenders for which they might require additional collateral. Regardless of the reason, however, it is important for older individuals to know that they are more likely to be denied credit.
Borrower’s Age in Credit Decisions
The Equal Credit Opportunity Act aims to “promote the availability of credit to all creditworthy applicants without regard to race, color, religion, national origin, sex, marital status, or age.” While the law prohibits discrimination on the basis of any of these factors, it does not prohibit lenders from using age as part of a credit scoring system. Credit scoring systems are divided into two types – judgmental and empirically derived.
Under a judgmental system, age-related factors can be considered in several specific circumstances. For example, “[a] creditor may consider the applicant’s occupation and length of time to retirement to ascertain whether the applicant’s income (including retirement income) will support the extension of credit to its maturity.” Or, “[a] creditor may consider the adequacy of any security offered when the term of the credit extension exceeds the life expectancy of the applicant and the cost of realizing on the collateral could exceed the applicant’s equity.” Or, “[a] creditor may consider the applicant’s age to assess the significance of length of employment (a young applicant may have just entered the job market) or length of time at an address (an elderly applicant may recently have retired and moved from a long-term residence).”
Under an empirically derived scoring system, age can also be considered. Specifically, the federal regulation preempts state law that “[p]rohibits asking about or considering age in an empirically derived, demonstrably and statistically sound, credit scoring system to determine a pertinent element of creditworthiness, or to favor an elderly applicant.”
The main takeaway is that applicant age and mortgage application outcomes may be correlated because lenders may consider age in connection with a relevant credit risk factor. Thus, finding a relationship does not necessarily imply that the creditor is violating the law. The question of whether such a correlation exists, nevertheless, remains important.
Data and Methodology
To determine the relationship between age and rejection of a mortgage application, this analysis uses data from the HMDA for 2018-2020. These data contain applicant and co-applicant age and a rich set of applicant, property, and loan characteristic variables, which makes it possible to control for observable characteristics that may affect lending decisions.
The analysis focuses on a subset of mortgage applications – namely, rate-and-term refinance applications that are associated with a single borrower. The focus on single-borrower stems from the need to know the borrower’s age, which is unclear when an application has two borrowers. The focus on refinance applications is driven by the goal to have as little selection bias as possible. The problem with home purchase loan applications is that no data are available on whether the borrower is a current homeowner, which is important because homeowners tend to have more financial resources and longer credit histories than renters.
The final sample contains approximately 5 million rate-and-term refinance applications, which make up 27 percent of total mortgage applications (see Figure 1); and borrowers who are older than age 50 account for about 40 percent of the rate-and-term refinance applications.
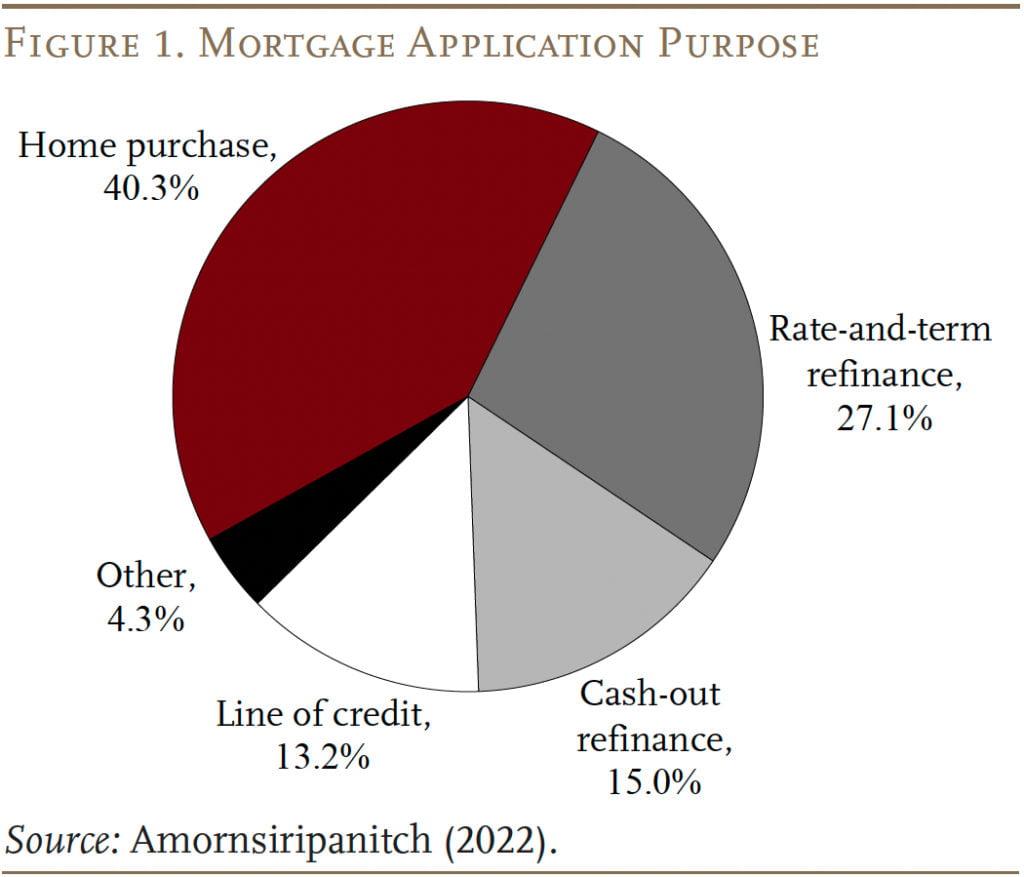
The relationship between applicant age and rejection probability is estimated using an ordinary least squares (OLS) regression equation:
Rejected = ƒ(age + applicant/loan/property characteristics + tract by month FE + lender by quarter FE).
Rejected is an indicator variable that equals one if the application gets rejected and zero otherwise. Applicants are sorted into the following age groups: 18-24, 25-29, 30-39, 40-49, 50-59, 60-69, and 70+. Applications associated with individuals ages 18-24 are used as the reference group. The equation also includes applicant, loan, and property characteristics – specifically, sex, race, ethnicity, credit score, income, loan-to-value ratio, debt-to-income ratio, loan features, property types, lien status, and approval under an automatic underwriting system. Finally, the equation includes month by census tract fixed effects and lender by year-quarter fixed effects.
This regression specification makes it possible to estimate the conditional correlation between applicant’s age and mortgage application outcomes among individuals who applied for rate-and-term refinance loans under very similar circumstances; that is, the applications were submitted to the same lender in the same month for properties in the same census tract.
Results
Figure 2 presents selected coefficients from the OLS regression that relates the probability of being rejected to age group and other factors. Several patterns are notable. First, compared to the reference group, the relationship between applicant age and probability of rejection increases monotonically with age. Second, the economic magnitude of these coefficients is large, given that the average rejection rate is 17.5 percent. Third, relative to race and ethnicity, applicant age is an equally important correlate of mortgage approval. Finally, the coefficient for female applicants is negative and statistically significant, suggesting that the probability of rejection is lower for women.
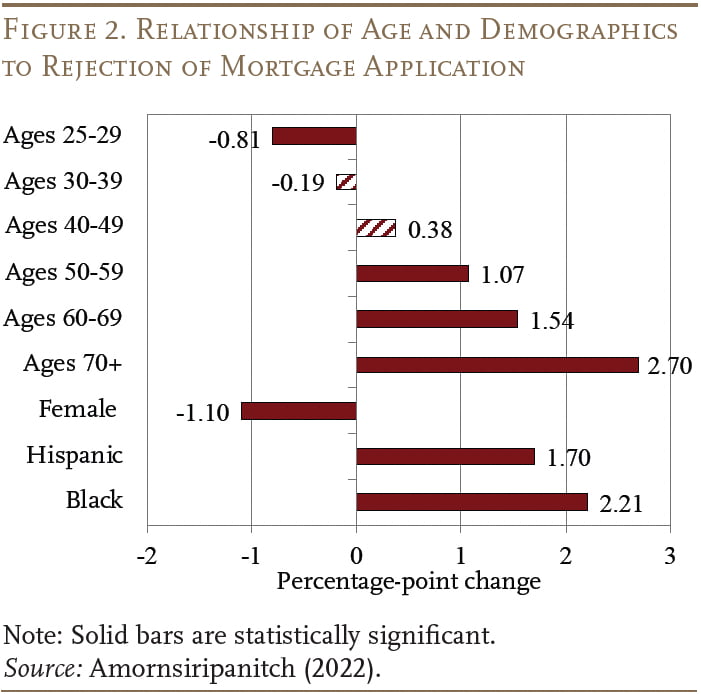
The results are robust. As noted, the equation includes lender by year-quarter fixed effects, which means that the findings are not driven by older individuals applying for mortgages with more stringent lenders. Excluding 2020 applications produces the same pattern, which means the results are not driven by COVID. Omitting age groups from the equation does not affect the coefficients on the other variables. Separate estimates for government guaranteed loans produce the same qualitative results.2These loans include those guaranteed by the Veterans Administration, the Federal Housing Administration, and the U.S. Department of Agriculture Farm Service Agency. Finally, the pattern is also evident for cash-out refinance applications.
Since the sample is so large, it is also possible to estimate the correlation between age and probability of rejection at each age and by sex. The results show that the marginal difference in rejection probability is not a step function but rather increases with each additional year of age and accelerates around age 70 (see Figure 3). Interacting the age and sex variables also shows that the rejection rate for women increases much more slowly with age than for men.
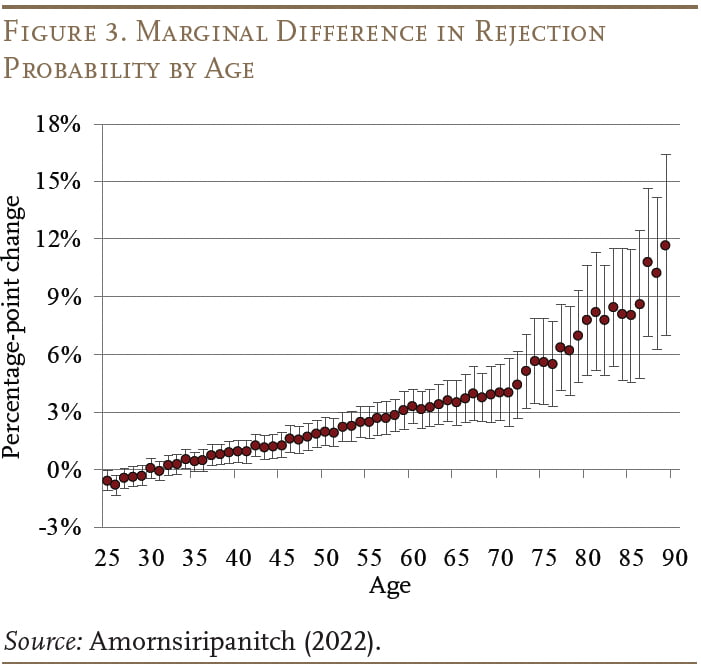
The bottom line is that whether the analysis focuses on age group or individual ages, the probability of rejection of an application increases with age. This result is surprising because credit score and wealth are positively correlated with age.
Possible Explanations
A starting place for explaining the link between age and rejection is the underwriters’ explanations for the decisions. To figure out which explanations relate to the rising rejection pattern with age, the equation can be re-estimated so that the dependent variable is a certain rejection reason. For example, the first reason for rejection in the data set is a high debt-to-income ratio. Hence, in the first equation, the indicator variable equals one if the application is rejected because its debt-to-income ratio is too high. The equation is subsequently re-estimated for each rejection reason.
The rejection reason that seems to qualitatively match the correlation between applicant age and application rejection is “insufficient collateral,” which means that the homeowner does not have enough equity on his or her property to take out the desired loan amount.3Another rejection reason that shows the same age pattern is the catch-all reason “other.” While this category could be capturing the effect of age-related incapacity to contract, the same quantification approach described above shows that “other” explains very little of the overall age effect on mortgage application rejection. One way to quantify the effect of insufficient collateral is to re-estimate the baseline age regression with a dependent variable that is equal to the original rejected indicator variable except for cases where the application was rejected due to insufficient collateral. The result of this exercise shows that insufficient collateral accounts for approximately 50 to 70 percent of the age effect.
The importance of insufficient collateral is consistent with earlier findings that older homeowners are less able to maintain the quality of their homes.4Campbell et al. (2011). Therefore, the value of their collateral may have dropped substantially between when they first bought the property and when they applied for refinancing. Insufficient collateral could also result if the loan amount has grown relative to the property value. This event could occur if the original mortgage has a negative amortization feature or the borrower wishes to consolidate multiple mortgages into one. Insufficient collateral is also consistent with older applicants who are forced to carry a mortgage into retirement being more financially distressed, as they may lack the funds to pay for adequate maintenance.
Factors other than those cited by the underwriter could also influence the pattern between age and rejection. As noted, it is possible that the sample used in the analyses includes a disproportionate number of older applicants who are in financial distress and therefore less credit-worthy. At the other extreme, applicants 18-29 could have higher-than-average credit quality compared to others in their age group or have older mortgage guarantors associated with the application. Together, these two effects are likely to cause the estimated age gap in rejection probabilities to be too large, relative to the true age gap.
Omitting an important variable that is related to creditworthiness and age could also lead to a relationship between age and rejections. Life expectancy or age-related mortality risk is the obvious example. Having a borrower die can be costly to the lender, because it increases the likelihood of the loan being paid off early (prepayment risk) or entering foreclosure (default and recovery risk). All else equal, this set of risks is higher for older borrowers than for younger borrowers (see Figure 4). Therefore, a rational and risk-averse lender should consider age-related risks when making lending decisions.
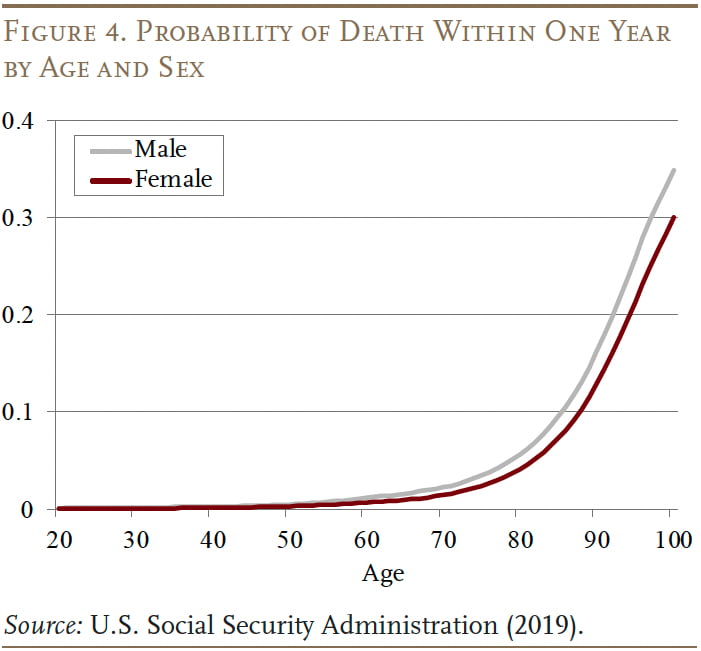
Indeed, several facts suggest that age-related mortality risk could be driving the correlations presented above. First, mortality risk, like the probability of rejection, generally increases with age. Second, the increase in rejection probability accelerates in old age, which is consistent with the fact that increases in mortality risk are much larger in old age. Third, the difference in rejection probability between men and women becomes larger in old age, which is consistent with the divergence in mortality risk between men and women in old age. Lastly, the insufficient-collateral explanation could be interpreted as lenders requiring the borrower to put up more collateral as age-related mortality risk increases.
In principle, it is plausible that taste-based age discrimination is a factor that is driving the rejection probability results. However, such discrimination is unlikely to produce a smooth increase in the probability of rejection as a person ages.
Conclusion
The relationship between age and rejection is large and robust. The equation includes lender by year-quarter fixed effects, which means that the findings are not due to older individuals applying for mortgages with more stringent lenders. Excluding 2020 applications produces the same pattern, which means the results are not driven by COVID. Omitting age groups from the equation does not affect the coefficients on the other variables. Separate estimates for government guaranteed loans produce the same qualitative results. Finally, the pattern is also evident for cash-out refinance applications.
The results do not necessarily indicate, however, that lenders are violating fair lending legislation. First, the regulations allow the consideration of a borrower’s age in credit decisions under some circumstances. Second, although the equation controls for a large set of observable characteristics, the results should be viewed as an association between age and rejection – not a causal relationship. Third, mortality risk has real economic implications for lenders for which they might require additional collateral. Regardless of the reason, however, it is important for older individuals to know that they are more likely to be denied credit.
References
Amornsiripanitch, Natee. 2022. “The Age Gap in Mortgage Access.” Working Paper.
Campbell, John Y., Stefano Giglio, and Parag Pathak. 2011. “Forced Sales and House Prices.” American Economic Review 101(5): 2108-2131.
U.S. Social Security Administration. 2019. “Period Life Table, 2019.” Washington, DC. Available at: https://www.ssa.gov/oact/STATS/table4c6.html
Endnotes
- 1Amornsiripanitch (2022).
- 2These loans include those guaranteed by the Veterans Administration, the Federal Housing Administration, and the U.S. Department of Agriculture Farm Service Agency.
- 3Another rejection reason that shows the same age pattern is the catch-all reason “other.” While this category could be capturing the effect of age-related incapacity to contract, the same quantification approach described above shows that “other” explains very little of the overall age effect on mortgage application rejection.
- 4Campbell et al. (2011).